Case study: How NY-Presbyterian has found success in not rushing to implement AI
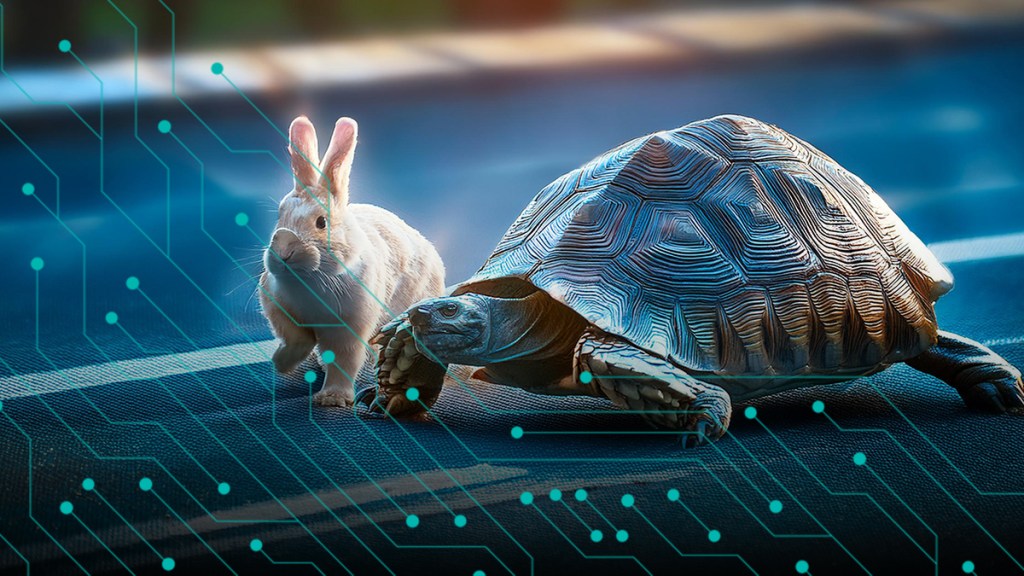
Join our daily and weekly newsletters for the latest updates and exclusive content on industry-leading AI coverage. Learn More
Leaders of AI projects today may face pressure to deliver quick results to decisively prove a return on investment in the technology. However, impactful and transformative forms of AI adoption require a strategic, measured and intentional approach.
Few understand these requirements better than Dr. Ashley Beecy, Medical Director of Artificial Intelligence Operations at New York-Presbyterian Hospital (NYP), one of the world’s largest hospitals and most prestigious medical research institutions. With a background that spans circuit engineering at IBM, risk management at Citi and practicing cardiology, Dr. Beecy brings a unique blend of technical acumen and clinical expertise to her role. She oversees the governance, development, evaluation and implementation of AI models in clinical systems across NYP, ensuring they are integrated responsibly and effectively to improve patient care.
For enterprises thinking about AI adoption in 2025, Beecy highlighted three ways in which AI adoption strategy must be measured and intentional:
Good governance for responsible AI development
A needs-driven approach driven by feedback
Transparency as the key to trust
Good governance for responsible AI development
Beecy says that effective governance is the backbone of any successful AI initiative, ensuring that models are not only technically sound but also fair, effective and safe.
AI leaders need to think about the entire solution’s performance, including how it’s impacting the business, users and even society. To ensure an organization is measuring the right outcomes, they must start by clearly defining success metrics upfront. These metrics should tie directly to business objectives or clinical outcomes, but also consider unintended consequences, like whether the model is reinforcing bias or causing operational inefficiencies.
Based on her experience, Dr. Beecy recommends adopting a robust governance framework such as the fair, appropriate, valid, effective and safe (FAVES) model provided by HHS HTI-1. An adequate framework must include 1) mechanisms for bias detection 2) fairness checks and 3) governance policies that require explainability for AI decisions. To implement such a framework, an organization must also have a robust MLOps pipeline for monitoring model drift as models are updated with new data.
Building the right team and culture
One of the first and most critical steps is assembling a diverse team that brings together technical experts, domain specialists and end-users. “These groups must collaborate from the start, iterating together to refine the project scope,” she says. Regular communication bridges gaps in understanding and keeps everyone aligned with shared goals. For example, to begin a project aiming to better predict and prevent heart failure, one of the leading causes of death in the United States, Dr. Beecy assembled a team of 20 clinical heart failure specialists and 10 technical faculty. This team worked together over three months to define focus areas and ensure alignment between real needs and technological capabilities.
Beecy also emphasizes that the role of leadership in defining the direction of a project is crucial:
AI leaders need to foster a culture of ethical AI. This means ensuring that the teams building and deploying models are educated about the potential risks, biases and ethical concerns of AI. It is not just about technical excellence, but rather using AI in a way that benefits people and aligns with organizational values. By focusing on the right metrics and ensuring strong governance, organizations can build AI solutions that are both effective and ethically sound.
A need-driven approach with continuous feedback
Beecy advocates for starting AI projects by identifying high-impact problems that align with core business or clinical goals. Focus on solving real problems, not just showcasing technology. “The key is to bring stakeholders into the conversation early, so you’re solving real, tangible issues with the aid of AI, not just chasing trends,” she advises. “Ensure the right data, technology and resources are available to support the project. Once you have results, it’s easier to scale what works.”
The flexibility to adjust the course is also essential. “Build a feedback loop into your process,” advises Beecy, “this ensures your AI initiatives aren’t static and continue to evolve, providing value over time.”
Transparency is the key to trust
For AI tools to be effectively utilized, they must be trusted. “Users need to know not just how the AI works, but why it makes certain decisions,” Dr. Beecy emphasizes.
In developing an AI tool to predict the risk of falls in hospital patients (which affect 1 million patients per year in U.S. hospitals), her team found it crucial to communicate some of the algorithm’s technical aspects to the nursing staff.
The following steps helped to build trust and encourage adoption of the falls risk prediction tool:
Developing an Education Module: The team created a comprehensive education module to accompany the rollout of the tool.
Making Predictors Transparent: By understanding the most heavily weighted predictors used by the algorithm contributing to a patient’s risk of falling, nurses could better appreciate and trust the AI tool’s recommendations.
Feedback and Results Sharing: By sharing how the tool’s integration has impacted patient care—such as reductions in fall rates—nurses saw the tangible benefits of their efforts and the AI tool’s effectiveness.
Beecy emphasizes inclusivity in AI education. “Ensuring design and communication are accessible for everyone, even those who are not as comfortable with the technology. If organizations can do this, it is more likely to see broader adoption.”
Ethical considerations in AI decision-making
At the heart of Dr. Beecy’s approach is the belief that AI should augment human capabilities, not replace them. “In healthcare, the human touch is irreplaceable,” she asserts. The goal is to enhance the doctor-patient interaction, improve patient outcomes and reduce the administrative burden on healthcare workers. “AI can help streamline repetitive tasks, improve decision-making and reduce errors,” she notes, but efficiency should not come at the expense of the human element, especially in decisions with significant impact on users’ lives. AI should provide data and insights, but the final call should involve human decision-makers, according to Dr. Beecy. “These decisions require a level of ethical and human judgment.”
She also highlights the importance of investing sufficient development time to address algorithmic fairness. The baseline of simply ignoring race, gender or other sensitive factors does not ensure fair outcomes. For example, in developing a predictive model for postpartum depression–a life threatening condition that affects one in seven mothers, her team found that including sensitive demographic attributes like race led to fairer outcomes.
Through the evaluation of multiple models, her team learned that simply excluding sensitive variables, what is sometimes referred to as “fairness through unawareness,” may not always be enough to achieve equitable outcomes. Even if sensitive attributes are not explicitly included, other variables can act as proxies, and this can lead to disparities that are hidden, but still very real. In some cases, by not including sensitive variables, you may find that a model fails to account for some of the structural and social inequities that exist in healthcare (or elsewhere in society). Either way, it is critical to be transparent about how the data is being used and to put safeguards in place to avoid reinforcing harmful stereotypes or perpetuating systemic biases.
Integrating AI should come with a commitment to fairness and justice. This means regularly auditing models, involving diverse stakeholders in the process, and making sure that the decisions made by these models are improving outcomes for everyone, not just a subset of the population. By being thoughtful and intentional about the evaluation of bias, enterprises can create AI systems that are truly fairer and more just.
Slow and steady wins the race
In an era where the pressure to adopt AI quickly is immense, Dr. Beecy’s advice serves as a reminder that slow and steady wins the race. Into 2025 and beyond, a strategic, responsible and intentional approach to enterprise AI adoption is critical for long-term success on meaningful projects. That entails holistic, proactively consideration of a project’s fairness, safety, efficacy, and transparency, as well as its immediate profitability. The consequences of AI system design and the decisions AI is empowered to make must be considered from perspectives that include an organization’s employees and customers, as well as society at large.